Powering AI Sustainably: Balancing Renewable Energy and Efficiency to Meet Growing Demands
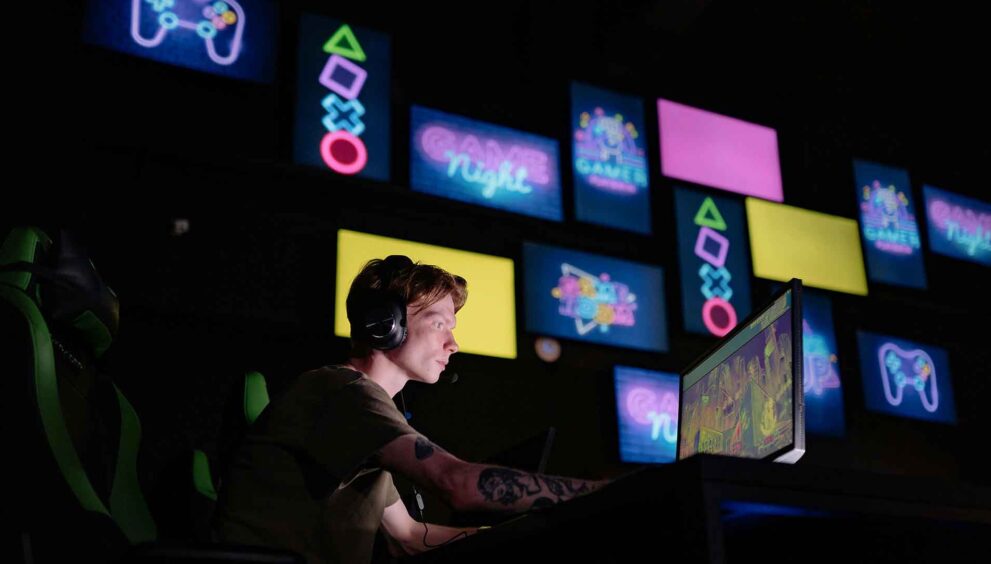
Artificial Intelligence (AI) has become an integral part of our lives, powering everything from recommendation engines to cutting-edge innovations in medicine and self-driving technology. But behind the breakthroughs lies a massive energy demand that’s putting a strain on our power infrastructure. Major tech companies like Google have seen their total greenhouse gas emissions climb nearly 50% in just five years, largely because of the electricity needed to support AI data centers. With AI’s demand for power projected to double by 2026, addressing this issue is both urgent and complex. In this article, we explore the environmental impact of AI’s energy consumption and examine whether a balanced approach between renewable energy and nuclear power could provide a sustainable solution.
The Escalating Energy Demands of AI
AI’s meteoric rise has come with substantial energy costs. Large language models and other forms of generative AI require extensive computational power, leading to high electricity consumption primarily from data centers. These centers house thousands of servers, each needing constant power and cooling to function. This enormous demand for energy is largely due to the resource-intensive nature of training and running AI models, especially as these systems continue to grow in complexity and scope.
The integration of AI into diverse sectors—finance, healthcare, retail, transportation, and beyond—only compounds the problem. As more industries adopt AI, the power required to support these systems will continue to skyrocket. Without intervention, AI’s growing energy demands could become unsustainable, causing an even more significant environmental impact in the coming years.
The Role of Renewable Energy
Renewable energy sources like wind, solar, and hydroelectric power have long been heralded as ideal solutions for reducing carbon emissions. These sources offer the potential to reduce AI’s environmental footprint by providing clean, low-emission electricity to data centers. Companies like Google and Microsoft have committed to powering their operations with 100% renewable energy, with Google claiming to have offset its carbon footprint entirely since 2007.
However, renewable energy also has limitations. Firstly, the rapid increase in AI’s energy demand may outpace the availability of renewables. While renewable sources are growing, the global energy infrastructure still relies heavily on fossil fuels, especially during peak demand periods or when natural conditions are less favorable for wind and solar power. Cloudy days, low wind speeds, or dry spells can all reduce the output of renewable energy sources, making it difficult to ensure a continuous power supply for AI-dependent data centers.
Secondly, renewable energy often requires large land areas for wind farms and solar arrays. As energy demands increase, the environmental impact of building and maintaining renewable infrastructure becomes more significant. This leads to a crucial question: Can renewables alone realistically meet AI’s exponential energy demands, or do we need to look at alternative solutions?

Nuclear Energy as a Low-Carbon Alternative
For many tech companies, nuclear energy is emerging as a viable, low-carbon option to meet high energy demands. Nuclear power offers a steady, reliable source of electricity, unlike renewable energy, which is often intermittent. The ability of nuclear power plants to produce large amounts of energy around the clock makes it especially well-suited to supporting the continuous operation of AI data centers.
Unlike coal or natural gas, nuclear energy generates minimal greenhouse gas emissions, making it an attractive option for companies focused on reducing their carbon footprint. Many countries, including France, Sweden, and Canada, already rely on nuclear energy as a significant part of their energy mix. Tech companies are exploring partnerships and investments in nuclear energy as part of their broader sustainability goals, recognizing its potential to provide a consistent power source without the emissions associated with fossil fuels.
However, nuclear energy has its own set of challenges, from high upfront construction costs to the long-term management of radioactive waste. Public perception of nuclear power is also a significant barrier. Despite advancements in nuclear technology, accidents such as those at Chernobyl and Fukushima continue to fuel skepticism. Overcoming these hurdles will require education, transparency, and investment in safer, more advanced nuclear technologies like small modular reactors (SMRs).
Balancing the Energy Mix: Efficiency and Hybrid Approaches
A balanced energy approach that combines renewable energy, nuclear power, and efficiency improvements may be the most practical path forward. Here’s how each element could contribute to a sustainable solution for AI’s energy demands:
- Efficiency Improvements: Enhancing the efficiency of AI models and data centers can help reduce overall energy consumption. This includes optimizing algorithms, using energy-efficient hardware, and improving data center designs to reduce cooling needs. Machine learning researchers are increasingly focused on creating models that achieve high performance with less computational power, a trend that could help mitigate the rapid increase in energy demand.
- Renewable Energy as a Core Component: Renewable sources should remain at the center of efforts to power AI systems sustainably. Despite limitations, wind, solar, and hydroelectric power are essential for reducing carbon emissions. Companies should continue investing in renewable energy infrastructure, particularly in regions with abundant natural resources.
- Nuclear Energy as a Stable Backup: To address the limitations of renewables, nuclear energy could provide a stable backup, ensuring that AI-powered systems receive a continuous power supply. Small modular reactors (SMRs) could play a critical role here, offering safer and more flexible nuclear power options that are suitable for various scales of operation.
- Hybrid Approach for Resilience: A hybrid approach that integrates renewable and nuclear energy sources may be the most resilient strategy. This approach allows companies to prioritize renewable energy while using nuclear power to fill gaps in supply, especially during periods of peak demand or when renewable sources fall short.
Case Study: Google’s Increasing Energy Consumption
Google’s recent increase in greenhouse gas emissions underscores the urgency of addressing AI’s energy demands. Despite ambitious sustainability goals, Google’s reliance on energy-hungry data centers has pushed its total emissions up nearly 50% over five years. This example highlights the difficulty of reducing emissions solely through renewable energy, particularly for tech giants with extensive AI infrastructure. Google’s case suggests that a hybrid energy approach could offer a more sustainable way forward, combining renewables with nuclear power to meet high energy demands without compromising environmental goals.
Conclusion: The Future of AI’s Energy Sustainability
The rapid advancement of AI brings incredible opportunities but also significant environmental challenges. Addressing AI’s energy consumption will require a multi-faceted approach, balancing the use of renewable energy with the reliability and low carbon emissions of nuclear power. Efficiency improvements in AI models and data center operations will also play a crucial role in managing energy demand.
In the end, there is no single solution to the environmental challenges posed by AI’s energy needs. By integrating renewable and nuclear energy sources, investing in efficiency, and remaining open to innovation, we can build an energy infrastructure that supports AI’s growth without sacrificing sustainability. This balanced approach offers a promising path to harness AI’s transformative potential while protecting our planet’s resources for future generations.
Digital marketing and corporate communications specialist. Graphic and web designer, video editor and content developer.